Control As Inference
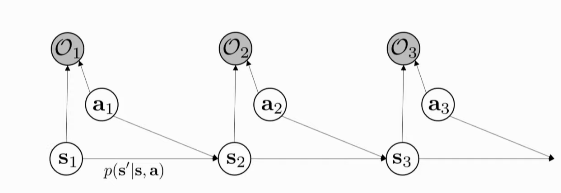
Figure 1: PGM for decision making for the first 3 time-steps
We introduce a binary variable for Optimality
If we choose
With this Probabilistic Graph Model, we can:
- model sub-optimal behaviour (important for inverse RL)
- can apply inference algorithms to solve control and planning problems
- provides an explanation for why stochastic behaviour may be preferred (useful for exploration and transfer learning)
Inference
- compute backward messages
- compute policy
, the policy of this model under assumption of optimality - compute forward messages
- useful for figuring out which states the optimal policy lands in, for the inverse RL problem (not used for forward RL)
Backward Messages
where we assume actions are likely a priori uniform. From these equations, we can get:
For
If we choose
For
In a deterministic transition setting, the log and exp cancel out. However, this otherwise results in an optimistic transition, which is not a good idea!
What if the action prior is not uniform? We can always fold the action prior into the reward!
Policy computation
It turns out the policy is just the ratio between the 2 backward
messages. Substituting
One can also add a temperature:
Forward Messages
same derivations as Hidden Markov Model!
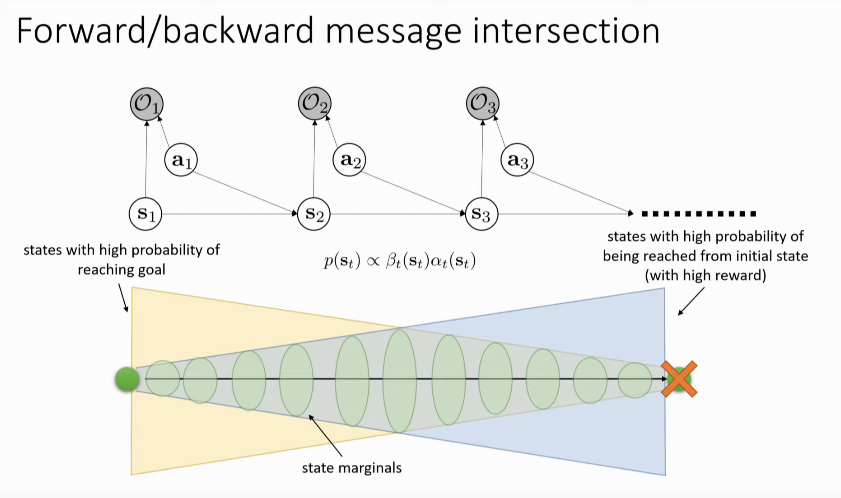
Resolving Optimism with Variational Inference
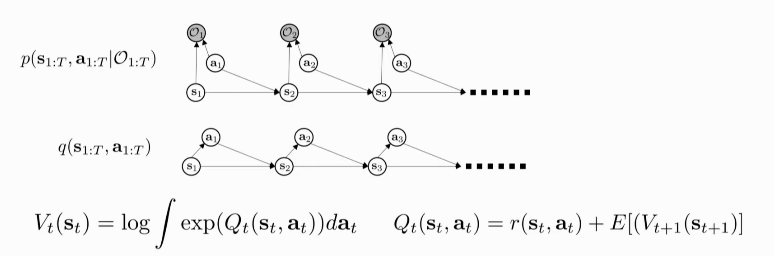
For more, see Levine, n.d..