XGBoost
XGboost is an end-to-end boosting system. It is sparsity-aware. (Chen and Guestrin, n.d.)
Regularized Learning Objective
For a given data set of \(n\) examples and \(m\) features, a tree ensemble model uses \(K\) additive functions to predict the output:
\begin{equation} \hat{y}_i = \phi(\mathbf{x_i}) = \sum_{k=1}^{K} f_k(\mathbf{x_i}), f_k \in F \end{equation}
where \(F\) is the space of regression trees, Each regression tree contains a continuous score on each of the leaf.
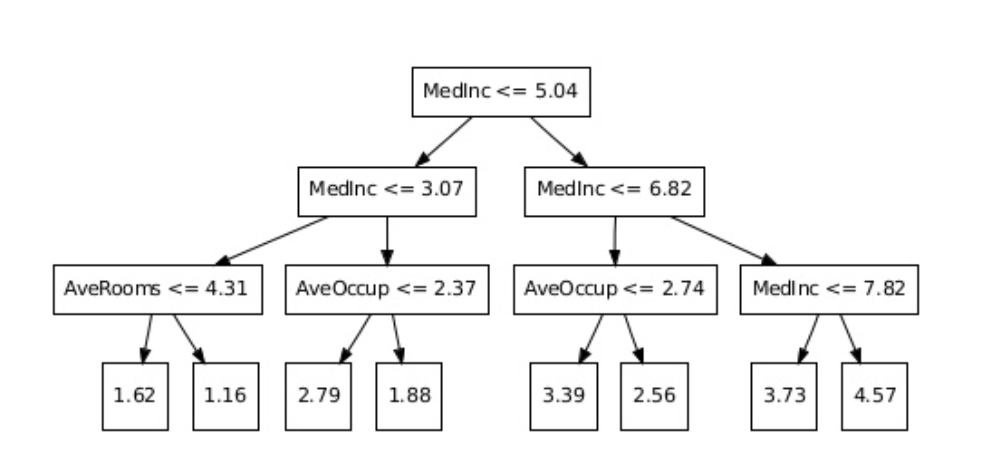
We wish to minimise the regularized objective:
\begin{equation} L(\phi) = \sum_i l(\hat{y}_i, y_i) + \sum_{k} \Omega(f_k) \end{equation}
where
\begin{equation} \Omega(f) = \gamma T + \frac{1}{2} \lambda \lVert w \rVert^2 \end{equation}
\(T\) being the number of leaves in the tree, and \(w_i\) being the ith weight of the leaf.
Gradient Tree Boosting
Since the regularized objective contains functions, the objective cannot be optimized in the Euclidean space.
Let \(\hat{y}_i^t\) be the prediction of the ith instance on the $t$-th iteration. We need to add \(f_t\) to minimize the following objective:
\begin{equation} L^{(t)} = \sum_{i=1}^{n} l(y_i, \hat{y}_i^{(t-1)} + f_t(\mathbf{x}_i)) + \Omega(f_t) \end{equation}
We can perform a Taylor expansion, and obtain:
\begin{equation} L^{(t)} = \sum_{i=1}^{n} l(y_i, \hat{y}_i^{(t-1)}) + g_i f_t(\mathbf{x}_i) + \frac{1}{2}h_i f_t^2(\mathbf{x}_i) + \Omega(f_t) \end{equation}
where \(g_i = \partial_{\hat{y}^{(t-1)}} l(y_i, \hat{y}^{(t-1)})\), and \(h_i = \partial^2_{\hat{y}^{(t-1)}} l(y_i, \hat{y}^{(t-1)})\) are the first and second order gradient statistics (gradients and Hessians). Removing the constant terms, at step \(t\), the objective is simplified to:
\begin{equation} \tilde{L}^{(t)} = \sum_{i=1}^{n} g_i f_t(\mathbf{x}_i) + \frac{1}{2}h_i f_t^2(\mathbf{x}_i) + \Omega(f_t) \end{equation}
If we define \(I_j = \{ i | q (x_i) = j\}\) as the instance set of leaf \(j\), then we can rewrite:
\begin{equation} \tilde{L}^{(t)} = \sum_{j=1}^T [(\sum_{i \in I_j} g_i) w_j + \frac{1}{2} (\sum_{i \in I_j} h_i + \lambda) w_j^2] + \gamma T \end{equation}
and we find that for a fixed structure \(q(x)\), we can compute the optimal weight \(w_j^*\) of leaf \(j\):
\begin{equation} w_j^{*} = - \frac{\sum_{i \in I_j} g_i}{\sum_{i \in I_j} h_i + \lambda} \end{equation}
This can then be used to score a tree structure \(q\). However, since enumerating all possible trees is computationally expensive, we use an algorithm to iteratively add branches to the tree, by computing the loss reduction from splitting:
\begin{equation} L_{\text{split}} = \frac{1}{2} \left[ \frac{\left( \sum_{i \in I_L} g_i \right)^2}{\sum_{i \in I_L} h_i + \lambda} + \frac{\left( \sum_{i \in I_R} g_i \right)^2}{\sum_{i \in I_R} h_i + \lambda} - \frac{\left( \sum_{i \in I} g_i \right)^2}{\sum_{i \in I} h_i + \lambda} \right] \end{equation}
Implementing Distributed XGBoost
The implementation of distributed XGBoost uses RABIT, and the Allreduce framework. XGBoost requires gradients and hessians from each distributed worker. This fit the allreduce framework, which broadcasts each worker’s reduce result across all processes. (Chen, Cano, and Zhou, n.d.)
Bibliography
Chen, Tianqi, and Carlos Guestrin. n.d. “Xgboost: A Scalable Tree Boosting System.” http://arxiv.org/abs/1603.02754v3.
Chen, Tianqi, Ignacio Cano, and Tianyi Zhou. n.d. “RABIT: A Reliable Allreduce and Broadcast Interface” 3 (2).